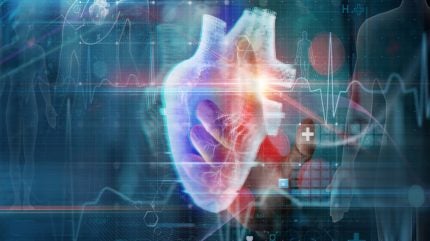
Research by the American Heart Association (AHA) estimates that 184 million Americans, approximately 61% of the population are expected to have some form of cardiovascular disease (CVD) by 2050, with an anticipated boom in costs with the indication set to inflict expenses of up to $1.8 trillion.
At the same time, the UK’s British Heart Foundation finds that there are currently 7.7 million people in the UK living with some form of CVD. Given these dire predictions, investment in the cardiac health space is rising.
In a period in which the acquisition of medical staff is both becoming harder and more necessary to run healthcare systems as is, healthcare service providers and medical device firms are looking to ensure critical services wherever possible. The promising prospect of AI automation could provide incremental efficiency gains which may, in turn, translate to better care outcomes.
Research by GlobalData estimates that the overall market for artificial intelligence (AI) sat at approximately $81.3 billion in 2022, whilst at the same time the market for remote patient monitoring sat at a value of around $600m, with that value estimated to rise to $760m by 2030. Previously in the Hospital 2040 series, we discussed how AI and automated systems can be used to better streamline hospital systems so as to better optimise workflow throughout the whole system.
Now, Hospital Management sits down with Stuart Long, CEO of the US-based virtual cardiac care firm InfoBionics, to understand how implementing AI-backed patient monitoring systems can keep a hospital’s cardiac care systems ahead of an impending wave of expected cases.
Joshua Silverwood: Given that so many American are expected to get some form of CVD, how do you see at home patient monitoring being important in that situation?
Stuart Long: I don’t think that at-home monitoring is a novelty anymore. I think in some sense we’re all doing some kind of home monitoring for the most part. Alost everybody in their home has some kind of device such as a blood pressure cuff or a thermometer. Also, Covid-19 really brought the idea home to roost.
How well do you really know your competitors?
Access the most comprehensive Company Profiles on the market, powered by GlobalData. Save hours of research. Gain competitive edge.
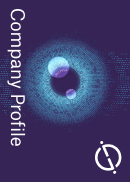
Thank you!
Your download email will arrive shortly
Not ready to buy yet? Download a free sample
We are confident about the unique quality of our Company Profiles. However, we want you to make the most beneficial decision for your business, so we offer a free sample that you can download by submitting the below form
By GlobalDataNow that the practice has reached some level of acceptance, we are still in the embryonic stages of it, but I think [remote monitoring] will become more ubiquitous across the board as we go on.
JS: Can you give me an idea as to how using AI and at home monitoring might cut down on hospital backlogs?
SL: I think if you look at what causes the backlog there’s a massive shortage in healthcare workers worldwide for providers. Even then, the population and the disease rates are outgrowing the pace at which companies are hiring people, not to mention just the added stress you have with healthcare providers where people are just choosing not to go that route. So, automation and the ability to assess things with machines, or at least through software that can assess information quicker and take away the mundane tasks of humans, is one of the things that’s really going to address it.
We will never replace humans in healthcare but in terms of jobs that don’t use this kind of technology, those jobs will become more scarce for sure. The idea of having artificial intelligence to be able to survey a large population of people autonomously and suggest where there is a problem, I think that will become quite a common practice. I think to some degree it already is that we just aren’t quite aware of it yet.
JS: What sort of guard rails do you think should be placed on these AI monitoring systems to keep them from hallucinating?
SL: If you think about AI in healthcare, there are three buckets. There is a place for generative AI, it’s in notetaking as well as the summarisation of clinical notes and things of that nature. Those are and probably will become much more highly trained on language models that are smaller than what we see these kinds of AIs trained on right now. Those are going to be attuned to businesses and managed in a way that the FDA will be able to have oversight and guidance.
The smaller and more coherent you can make the AI from a generative standpoint, with smaller language models that are tuned for it in actual practice, or a very specific workflow, the fewer and fewer hallucinations you get when you move into the world of clinical AI and workflow efficiency AI.
Those are really the two big, major buckets in healthcare. When you think about using AI in healthcare you think ‘Oh, it’s going to go whizz bang, it’s going to do something amazing’. You think it’s going to do something in clinical practice like finding diseases sooner or looking for hidden information in clinical documentation. The other bucket is how can we use AI to improve workflow and efficiency so as to reduce the backlog of patients waiting for care.
What we do [InfoBionic] specifically as medical device manufacturers is we take clinical data from a human and we run it through a system called signal processing. It doesn’t sound like AI but it’s a form of AI which cleanses the data and identifies what’s in it. We tell the signal processor to look for one specific thing and then we train multiple signal processors to look for multiple things and then you can combine them and look for a variety of different markers.
So our AI system can differentiate between electricity from the top of the heart, and from the bottom of the heart. From that perspective, you can then take machine learning and now that you have identified all the problems and cleaned them up, you can feed the AI ten thousand studies that are normal or abnormal and everything in between and teach an AI or algorithm to understand one particular arrhythmia. What’s normal, what’s abnormal what’s mediocre. Once we have an algorithm trained, we will sit down with clinicians who are blinded to whether it’s a real person or an AI doing the process and we will use that to determine the success of the algorithm before we submit it to the FDA.
JS: How might AI assisted cardiac systems improve outcomes for stroke patients?
SL: This gets into one of the other big buckets for AI, that is the clinical side of AI. Stroke is the end result of a disease that started a long time ago. What happens with a stroke is that you have plaque build-up in your heart and atrial fibrillation is one of the most common root causes of strokes or plaque build-up. Being able to identify atrial fibrillation, either as it’s started or very, very early or even finding it long before it was ever going to start, is important.
Where AI is helping today is that we are using monitors, which is a problem as people don’t tend to get a monitor until the problems have started, so we get a start earlier that allows us to identify atrial fibrillation even if someone might not have signs of arrhythmia going on when you just normally monitor it. We can detect subtle, early signs of flutter or atrial fibrillation and there are correlating clinical conditions that are combined with the AI to suggest that an event might happen.
I think in the very near future we will be able to look at a person’s electrocardiogram (ECG) results and, even if they’re not symptomatic, we can look at their records and assign a risk ratio. We will be able to say with a high degree of confidence that someone is going to develop atrial fibrillation and we can start treatments today that would help offset that. That is somewhere AI is going to help the most.
JS: Can you give me an idea of the cost benefit of implementing AI systems?
SL: I mean, it is across the board I think. The earlier you can detect anything, the more money you’re going to save, that’s, you know, that’s definitive. It has been proven time and time again that the cost to treat somebody who’s had a stroke is substantially more expensive than the cost if we found it before they ever had a stroke. Even if the disease has progressed to a point where they may not recover you have to do a more expensive intervention. It’s still far less expensive than treating somebody who’s had a stroke, ultimately.
It certainly will save money for the patient in the long run, because of medications or clinical events that they wouldn’t have to go through.