
The Accelerated Capability Environment (ACE) has collaborated with Kettering General Hospital (KGH) to develop a proof of concept (PoC) using artificial intelligence (AI) to improve patient bed allocation decisions in hospitals.
The PoC aims to ensure patients are in the right beds at the right times, and assist staff in making the best bed allocation choices.
The virtual hospital environment PoC is modelled on key KGH wards and was created with the help of ACE’s Vivace supplier community and the NHS England AI Lab.
It uses historic admission data to predict demand and generate bed suggestions for patients from the emergency department, and explains its recommendations to build trust in the AI tool.
The AI tool maintains human involvement through an explainability or human-in-the-loop approach, allowing schedulers to make the final bed allocation decisions.
This PoC claims to offer benefits such as more efficient bed scheduling using machine learning optimisation, improved decision-making with better data, reduced bed moves, and improved patient outcomes with cost reductions.
How well do you really know your competitors?
Access the most comprehensive Company Profiles on the market, powered by GlobalData. Save hours of research. Gain competitive edge.
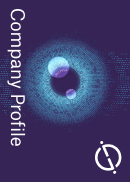
Thank you!
Your download email will arrive shortly
Not ready to buy yet? Download a free sample
We are confident about the unique quality of our Company Profiles. However, we want you to make the most beneficial decision for your business, so we offer a free sample that you can download by submitting the below form
By GlobalDataFuture steps include supporting multiple admissions, transfers, and discharges, integrating with existing hospital systems, and expanding to cover all specialities, wards, and bed types.
ACE recently collaborated with the NHS’s AI Skunkworks to develop an AI tool for faster Parkinson’s Disease diagnosis, expediting research by months, or even years.
The tool automatically identifies areas of interest in digitised brain images, reducing diagnosis time, and recorded a 92% accuracy rate.
It is claimed to have the potential for real-world applications and future use in differentiating disease stages and identifying dementia indicators in brain scans of live patients.